In the realm of technological innovation, the emergence of digital twins has reshaped how industries operate. These virtual replicas of physical assets or systems have become pivotal in optimising processes, predicting outcomes, and driving efficiency.
We aim to address misconceptions about the use of digital twins in renewable energy asset performance management through a blog series. Our blog series starts with explaining what a digital twin is and why it matters for managing renewable energy assets. Then, we'll show you how to make your plant work better using digital twins. And in the last post, we'll share some helpful tips and lessons we've learned from using SynaptiQ's digital twin technology based on real-world experiences.
What is a digital twin?
Imagine you have this cool idea for a new gadget, like a smart thermostat. Before you even build it, you create a digital twin of it. This digital twin serves as a sandbox for experimentation, enabling you to refine and fine-tune its functionality across various scenarios without the need for a tangible prototype. You can test it out, tweak it, and see how it works in different situations, all without even touching a physical prototype. Whether rendered as an intricate 3D model for immersive visualization or as a software-driven test bed facilitating seamless integration with interconnected devices, it provides a dynamic platform for iteration and enhancement.
Now, when you finally do build the real thermostat, the digital twin doesn't disappear. It sticks around, almost like a shadow, but in the digital realm. This twin helps you to verify the manufactured device mechanically versus the 3D model of the digital twin, or you could use the software model digital twin to verify your thermostat software behavior.
If there's a problem, you can simulate fixes on the digital twin before implementing them on the actual device. But the usefulness of digital twins doesn't stop there. They're not just for gadgets; they can be for entire systems or processes. For example, imagine a city's transportation network. You can create a digital twin of it to predict traffic flows, optimize routes, and even simulate emergencies like accidents or road closures.
So, digital twins aren't just about making virtual copies. They're powerful tools that span the entire lifecycle of something, from idea to disposal. And they're not just for after something's built; they can help shape and improve things from the very beginning. It's like having a twin that's always there to lend a helping hand, but in the digital world.
There are four sub-categories of digital twins that exist to support lifecycle management of products/assets:
- Pre-Digital Twin (PDT): Before the real thing even exists, there's this stage where you're planning and designing. That's where the Pre-Digital Twin comes in. It's like a blueprint or a virtual prototype of what you want to create. You use it to test ideas, make changes, and see how everything might work before you start building.
- Digital Model (DM): Once you've got the plan sorted out and you're ready to start building, you create a Digital Model. This is a detailed digital representation of the physical thing you're making. It's like a 3D model on a computer, showing exactly how every part fits together and how it should function.
- Digital Shadow (DS): Now, imagine you've built the real thing, like that smart thermostat we talked about earlier. The Digital Shadow is like a digital reflection of a real device. It's always connected to the physical thing, gathering data and monitoring its performance in real-time. If there's a problem with the real thermostat, the Digital Shadow helps figure out what's wrong and how to fix it.
- Digital Twin (DT): This is the big one. Digital twins are like the ultimate digital clone of the real thing. It's so alike that it can almost stand in for the physical device itself. You use it for all sorts of things, like testing, predicting how the real thing will behave in different situations, and even simulating changes or upgrades before you make them in real life.
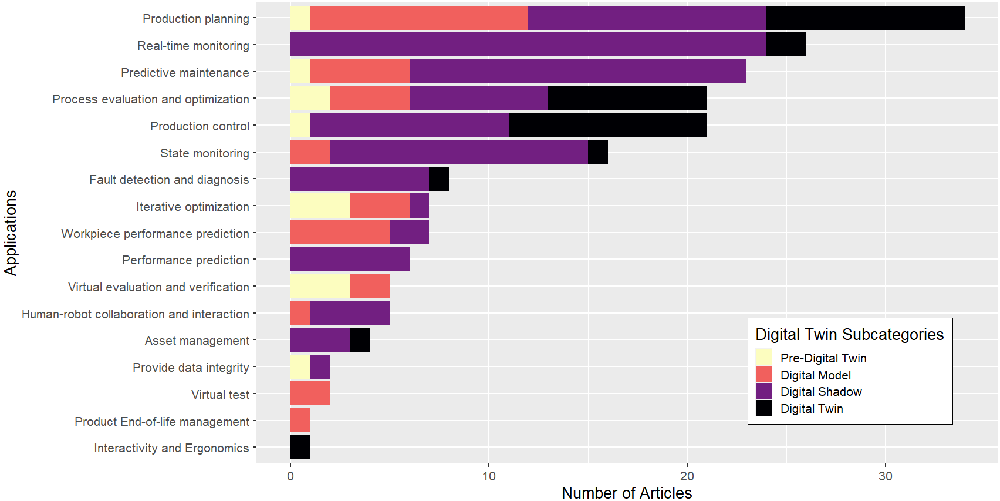
As seen in this figure, applications focused on monitoring and control present a large majority of the use of DS (real-time monitoring 93%, production control 47%, and state monitoring 80%), due to the lack of necessity for a command loop in these applications, whereas a larger proportion of DT are used in applications focused on optimisation (production planning 29%, process evaluation, and optimisation 35%).
Enhancing operational efficiency with digital twin: monitoring, analysis, and action
In general, virtual models can be employed for monitoring, analysing, and optimising the performance of their physical counterparts. Broadly, we can categorize their functions into three groups:
- Monitoring: Basic-level digital twins monitor using data from sensors and devices, visualizing the situation through a software program.
- Analysis: Through advanced algorithms and analytics, the digital twin assesses patterns, trends, and correlations within the data. This analytical process aims to derive valuable insights into the performance, efficiency, and potential issues of the physical counterpart
- Action: Advanced-level digital twins use intelligent algorithms to learn from collected data, identify issues, propose multiple solutions for each problem, and select the most suitable one.

Black Box vs. White Box approaches to operational digital twin development
In digital twin development, two main approaches stand out. These are the black box and the white box methods.
The black box method is a purely statistical approach. It relies on operational monitoring data and applies machine-learning techniques to create a statistical model of asset behaviour. This method involves fitting and correlating actual behaviour under various operational conditions. It provides robust and reliable results, thanks to the rapid advancement of artificial intelligence.
Alternatively, the white box method is physics-based. It is typically used in the design and engineering phase and utilizes a simulation model to predict asset behaviour under actual operating conditions. Such a simulation model typically consists of physical formulas that define the processes applicable to the system.
It is also possible to combine both techniques, to create an even more accurate digital twin of an asset’s expected behaviour. This is a concept known as grey-box modelling. Benefits of utilizing a digital twin that integrates physics, data, and AI utilizing a digital twin that integrates physics, data, and AI offers numerous advantages, particularly in the renewable energy sector. That’s why at 3E, for several important reasons, we advocate for the use of both white-box (physics-based) and grey-box (hybrid) modelling approaches:
- Firstly, a pure black box approach requires a training period. This can significantly delay the assessment of asset performance. In the case of solar, this could mean waiting at least two seasons or six months before gaining valuable insights.
- Secondly, the statistical approach will always consider a training period as a reference period, during which it is assumed that the plant will operate as expected. This unfortunately rarely reflects real-world scenarios. Meaning these approaches are always likely to overlook a number of performance losses.
- Also, the black box approach lacks the versatility to be considered a true digital twin, as it only serves the operational phase of an asset. In contrast, the white box and grey box approaches cover all the different lifecycle phases.

How the physics-based approach contributes to better decision-making and resilience
In multiple ways, the physics-based digital twin approach contributes to proactive decision-making and the overall resilience of renewable assets. This approach provides high-granularity time-series data on the expected behaviour of an asset at different component levels. This high-granularity dataset can be combined with high-quality monitoring data, and artificial intelligence techniques, to achieve incredibly robust and detailed failure root cause identification in operational systems. Compared to the machine learning approach, the physics-based approach offers improved early fault detection, identifies a higher number of loss categories, and more accurately quantifies losses. In doing so, this:
- Enhances transparency on asset performance among operational stakeholders.
- Facilitates more efficient decision-making and reporting.
- Enables proactive corrective actions to be taken, resulting in substantial long-term performance enhancements.
Physics based digital twin for lifecycle optimisation of renewable assets
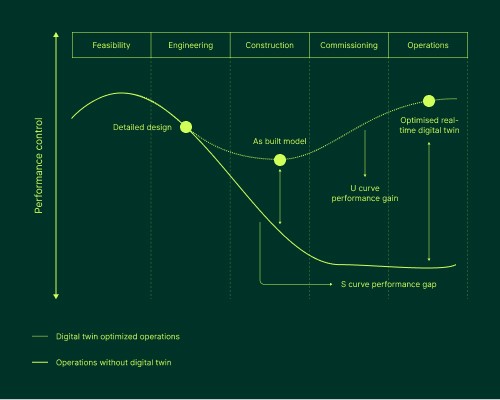
In the lifecycle of a typical renewable asset, several phases precede its operational state, each crucial for its success. The integration of digital twins significantly amplifies performance gains when implemented from project inception.
- Feasibility: Elevating performance stands as the linchpin for securing investment approval. Highlighting the potential performance gains underscores the project's viability and attractiveness to investors.
- Engineering: Balancing performance and cost optimisation is paramount during engineering phases. Outsourcing to third-party Engineering, Procurement, and Construction (EPC) firms risks diluting control over performance targets. A meticulous approach to digital twin implementation maintains a firm grip on performance metrics and enhances cost-effectiveness.
- Construction: Despite meticulous planning, construction often unveils unforeseen practical constraints that may compromise performance objectives. Persistent digital twin modeling throughout construction bolsters adherence to performance targets by swiftly addressing emerging challenges.
- Commissioning: Traditional as-built documentation and handover processes can lead to vital asset information loss. Rigorous digital twin integration halts this drain and facilitates the application of yield modeling, offering a robust performance benchmark under actual operating conditions.
- Operations: The operational phase presents an opportunity to refine performance insights. However, with a digital twin in place, the baseline for performance is significantly elevated.
The figure above clearly illustrates that embracing digital twins from the outset in Independent Power Producers (IPP) and Operations & Maintenance (O&M) for asset lifecycle management yields a distinctive U-shaped performance gain once the site is operational. Conversely, neglecting such integration can result in an S-shaped performance gap that accumulates over time without the optimised asset management facilitated by digital twins.
Stay tuned for the next installment of this blog series, where we delve into optimising renewable asset performance through precise and effective implementation of digital twins.